Translate this page into:
Comparative analysis of double inversion recovery (DIR) and diffusion tensor imaging (DTI) sequences for the detection of brain white matter diseases
*Corresponding author: Mr. Shubham Gupta, Department of Radiological Imaging Techniques, College of Paramedical Sciences, Teerthanker Mahaveer University, Moradabad, Uttar Pradesh, India. shubham.gupta5555@gmail.com
-
Received: ,
Accepted: ,
How to cite this article: Kumar D, Rastogi R, Diwakar SK, Gupta S. Comparative analysis of double inversion recovery (DIR) and diffusion tensor imaging (DTI) sequences for the detection of brain white matter diseases. Future Health. 2024;2:194-205. doi: 10.25259/FH_67_2024
Abstract
Introduction
Double inversion recovery (DIR) and diffusion tensor imaging (DTI) are important in the study of cerebral white matter diseases. DIR is more sensitive to cortical lesions, like those of multiple sclerosis, whereas DTI provides information about white matter microstructure. The specific contribution and limitations of each technique must be known for their appropriate application at the bedside.
Material and Methods
We have undertaken a comprehensive review of studies on DIR and diffusion imaging with resampling of orthogonal planes methodologies. Data were extracted from reliable databases such as Scopus, PubMed, and Web of Science. The analysis compared the diagnostic accuracy and clinical implications of these techniques. Using machine learning, we were able to manage the multidimensional datasets successfully. Much as this technology showed promising results in improving diagnostic outcomes by easing the analysis of complicated imaging data, we took a holistic approach that gave us the vantage point to assess the strengths and limitations of DIR and DTI methodologies against medical imaging.
Results
Recent research has supported the efficacy of DIR for the detection of cortical lesions in multiple sclerosis (MS) patients. DTI has been known to detect microstructural changes within white matter tracts. New imaging technologies, including AI image generation and machine learning algorithms, have significantly enhanced diagnostic power.
Conclusion
Integrating DTI with DIR has proven to be a very strong technique in white matter disease assessment, demonstrating better detection of lesions and microstructural changes. DTI is helpful in predicting clinical outcomes and helps with rehabilitation strategies. Advanced next-generation imaging techniques and machine learning algorithms improve diagnostic accuracy. Hence, continued research toward refining imaging modalities is needed to understand the precise role of white matter abnormalities in cognitive function. Future research directions in imaging for the detection of white matter diseases would come with the integration of advanced techniques, such as b-tensor encoding schemes in diffusion-weighted MRIs with machine learning.
Keywords
Double inversion recovery
diffusion tensor imaging
white matter disease
neuroimaging
comparative analysis.
INTRODUCTION
Double inversion recovery (DTI) and diffusion tensor imaging (DIR) sequences are contrasted, as both have very different roles in the assessment of brain pathologies. DTI will help in assessing microstructural changes in white matter tracts and hence give information about neural connectivity and integrity. On the other hand, DIR sequences have been proven to be highly sensitive in lesion and abnormality detection in the brain, particularly in pathologies such as MS and epilepsy. They have a higher rate of lesion detection compared to conventional sequences like fluid-attenuated inversion recovery (FLAIR) and T2. Moreover, synthetic DIR images have been proven to exhibit higher contrast to synthetic T2W-FLAIR and conventional DIR images, thus proving the advantage of DIR sequences in clinical neuroimaging. While DTI takes a look at microstructural changes, DIR sequences are much better at detecting and characterizing lesions; thus, the two techniques complement each other as tools for comprehensive assessment of brain imaging. DIR and DTI sequences are just two of the sequences used in detecting brain white matter diseases [Figure 1]. DIR is a pulse sequence for increasing the visibility of gray matter lesions, especially cortical lesions, in patients with MS.1 It has been shown to be superior to FLAIR in detecting lesions at different locations such as the cortical, periventricular, and infratentorial regions.2 DTI is a technique that measures water molecule diffusion in the brain tissue, giving information about white matter tract integrity. It has been used frequently to assess diseases of white matter, like MS.3
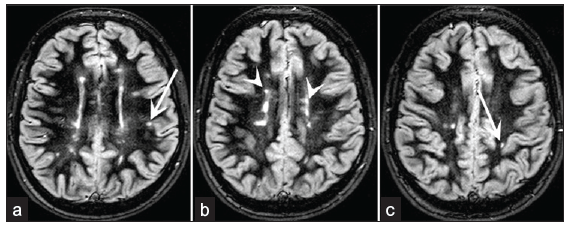
- Shows the Transverse Section of brain. (a-c) axial section of the supratentorial brain in the diffusion weighted imaging (DIR) Sequences with background suppression appears with the characteristics feature. The inflammatory lesion show sharper demarcation, thus better visibility. Although the contrast between lesion and gray matter seems very subtle, the DIR sequences image tends to be very sensitive in detecting juxtacortical and mixed white-gray matter lesions clearly shown by arrows and arrow heads. Besides, it is easily distinguished between juxtacortical and lesion with the help of the DIR sequences.
While DIR and DTI show some encouraging results in the detection of brain white matter disease, more studies are required with respect to the diagnostic accuracy of both techniques and their potential role in clinical practice.4
The white matter is composed of myelinated axons, oligodendrocytes, and glial cells. The oligodendrocytes produce myelin sheaths which envelop the axons and give the white matter its color [Figure 2]. These myelin sheaths ensure efficient conduction of electrical signals between different areas of the brain [Figure 3].5
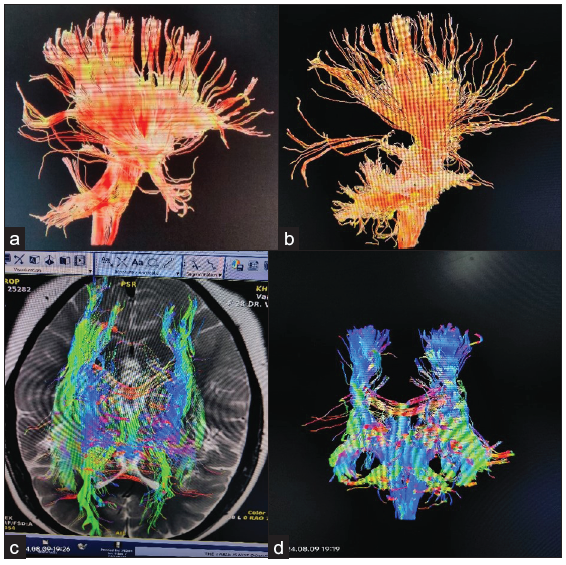
- Demonstration the Diffusion Tensor Imaging of white matter tract. (a and b) 3D Sagittal plane of brain nerve fibers shows the detailed anatomy of white matter tract. (c) T2W Axial and (d) Coronal respectively of brain white matter tract shows the connectivity structure of the brain. Bright colors indicate the directionally and integrity of the fiber bundles, indicating the neural communication and connectivity patterns.
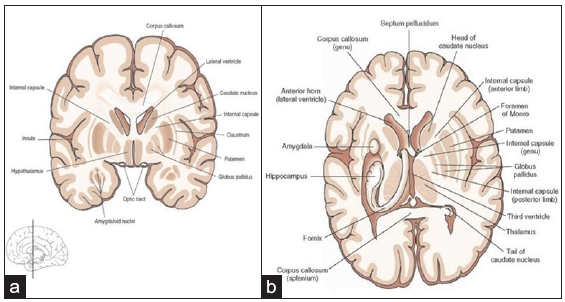
- (a) Coronal view and (b) Transverse view of the brain. It consists of myelinated axons, oligodendrocytes, and glial cells; the myelin sheath, produced by the oligodendrocytes, wraps the axons, giving the white matter its color. Myelin sheaths increase velocity and efficiency in the transmission of electrical impulses between different regions of the brain.
The white matter acts as a network of pathways that connect different regions of the central nervous system (CNS).5,6 It allows for the transmission of signals from one gray matter area to another, ensuring the timely delivery of information.5 The myelinated axons in the white matter are well-designed to conserve energy while maintaining this high-fidelity signal delivery.7 The white matter also contributes to the coordination of signal arrivals to target neurons and their summation with other synaptic inputs. The tightness of internal structural connectivity within white matter may impact the structure-function relationship as shown in Figure 4.
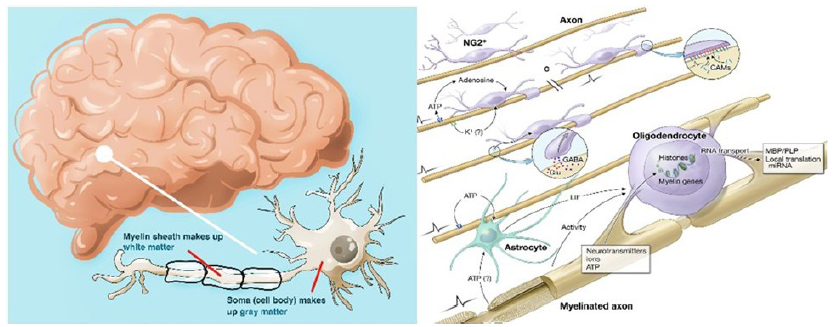
- Microstructural view of brain tissue revealing nerve fibers intricately woven amidst supportive astrocytes. The oligodendrocytes form myelin sheaths that envelop the nerve fibers, thus enabling fast transmission. The given tight view shows just how complicated the interplay is between neurons and glial cells, which is fundamental for brain function and communication.
Methodologies of Double Inversion Recovery (DIR) and Diffusion Tensor Imaging (DTI)
Data collection process
First, general information was obtained using keywords for an initial search for articles within databases like PubMed, ResearchGate, Scopus, Google Scholar and IEEE Xplore. These keywords such as DIR, DTI, white matter disease, neuroimaging, and comparative analysis, were useful in the search process. Search results: The first search resulted in 1,253 records. This was done to provide a very broad catch of studies in the several focus areas of this review.
Refining the search results: The original search returned 1,253 records. This was done to ensure that there is an extremely broad, catch-all of studies in the various focus areas of this review. As done in other reviews, the number of records were to journal articles only. This enhanced the relevance and quality of literature and brought down the number of records to 544.
Supplemental sources: A supplementary database search yielded 13 records. This was supplemented by sources, including citations in relevant articles, manual searching, and expert recommendations.
Screening and Eligibility
Removing duplicates: The duplicate records identified after the aggregation of results from various sources were removed. At this step, 487 unique records remained eligible for further screening.
Exclusion criteria: The titles and abstracts were screened for relevance, and only those of interest were included. The main exclusion criteria were as follows:
Non-imaging studies: 75 records
Records not obtained: 11 records
After the screening, 86 records were eliminated, and the full text of 401 records was selected for review.
Full-Text Review and Final Selection
Eligibility assessment: Each of the 401 articles were assessed based on the following eligibility criteria:
Relevance to focus areas: DIR, DTI, white matter disease, neuroimaging, comparative analysis
Availability of sufficient data to conduct comparative analysis
Robustness of methodology and quality of the study
Exclusion of full-text articles: 344 full texts were excluded in the review process review because they did not extend satisfactory coverage to focus areas. They had to be discarded to maintain the relevance and quality of the review.
Final inclusion: The final set of articles included in the review consisted of: 44 original articles, 5 book chapters, 3 conference articles, 1 thesis: 1, 1 case report, 3 review and meta-analysis articles: Diffusion-weighted magnetic resonance imaging (DW-MRI) is a non-invasive technique that can detect neuropathology in neural tissue as shown in Figure 5. Tensor-valued diffusion encoding schemes (b-tensor) have been developed to enhance the microstructural data obtained via DW-MRI, making it more specific to microstructural properties. Machine learning methods are useful for analyzing multidimensional data sets. In one study, b-tensor encoded DW-MRI data analyzed with machine learning showed potential for diagnosing histopathology and neurodegeneration.8,9 Another study proposed a disentangled conditional diffusion model for multi-contrast brain MRI super-resolution, which improved the quality of low-resolution contrasts by leveraging complementary information from multi-contrast MRI.10 Structural MRI image registration onto diffusion-weighted imaging variants was also investigated for tractographic samplings of the visual system, showing that structurally-placed ROIs with registration onto readout-segmented volumes were most suitable for white matter template generation.11 Lastly, SI characteristics derived from DWI_b1000 and T2-weighted-based MRI demonstrated excellent performance in differentiating white matter lesions caused by MS and cerebral small vessel disease.12
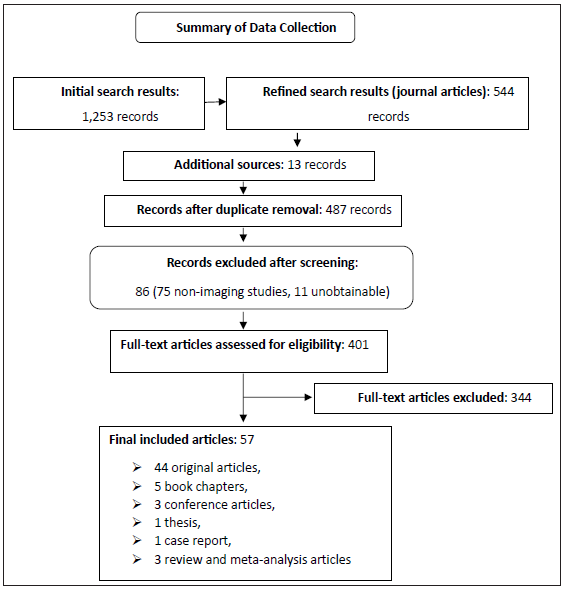
- Preferred reporting items for systematic reviews and meta-analyses flow chart for selection of the studies.
DIR and DTI are valuable techniques for detecting brain white matter disease. DIR, is effective in identifying cortical lesions in multiple sclerosis patients.13 On the other hand, DTI, provides insights into white matter integrity and microstructural changes associated with aging, Alzheimer’s disease, and cerebrovascular disease.14,15 DTI excels in characterizing tissue microstructure and detecting alterations related to brain vascular anomalies.16 On the other hand, DIR is particularly effective in pinpointing cortical lesions, especially in MS patients.14 DTI measures parameters such as fractional anisotropy (FA), mean diffusivity (MD), axial diffusivity (AD), and radial diffusivity (RD) to provide detailed information on microstructural changes.14,17 Neurite orientation dispersion and density imaging (NODDI) and mean apparent propagator (MAP) MRI offer alternative models for better characterization of microstructural changes related to aging and Alzheimer’s disease.18 Integrating DTI and DIR can offer a comprehensive assessment of brain white matter diseases, leveraging the strengths of each modality for a more thorough evaluation of white matter integrity and cortical lesion detection.19 DTI captures water diffusion in tissues, which is vital for studying brain connectivity. It utilizes Echo planar imaging (EPI), typically with short echo times (TE) and long repetition times (TR), and requires multiple diffusion directions and b-values. Motion correction and tensor calculations ensure accurate results. DIR suppresses specific tissue contrasts to enhance pathological visibility. Implemented with gradient echo (GRE) or fast spin echo (FSE), it utilizes long TR with two inversion times (TI) to nullify selected tissues. Optional fat saturation and motion correction techniques improve image quality. Both protocols [Table 1] offer insights into brain structure and pathology in clinical and research settings.
Sequence | DTI | DIR |
---|---|---|
Purpose | Study diffusion of water molecules in biological tissues, assess microstructural organization of white matter tracts | Suppress specific tissue contrasts, enhance visibility of certain pathological features or tissue types |
Sequence type | Echo Planar Imaging (EPI) | Gradient Echo (GRE) or Fast Spin Echo (FSE) |
FOV | 220-240 mm | 220-240 mm |
Slice thickness | 2-3 mm | 3-5 mm |
Matrix size | 128 × 128 or higher | 256 × 256 or higher |
NEX | 1 | 1 |
TE | Short, 60-100 ms | Short for GRE: 20-30 ms, Long for FSE: 80-100 ms |
TR | Long, 3000-10000 ms | Long, 3000-6000 ms |
Diffusion directions | Minimum 6 non-collinear directions, up to 64 for higher angular resolution | N/A |
B-values | b = 0 and b = 1000 s/mm2 | N/A |
Slice orientation | Axial, coronal, or sagittal | Axial, coronal, or sagittal |
Optional parameters | Gradient Strength, Partial Fourier Factor, Parallel Imaging | Fat Saturation, Parallel Imaging, Motion Correction |
Special considerations | Motion Correction, Eddy Current Correction, Tensor Calculation | TI Adjustment, Image Fusion |
DTI: Diffusion tensor imaging, DIR: Double inversion recovery, FOV: Field of view, NEX: Number of excitation, TE: Echo time, TR: Repetition time, N/A: Not available, TI: Time of inversion
COMPARATIVE ANALYSIS
The comparison between DTI and DIR, as depicted in Table 1, points out the differences in their purpose, sequence parameters, and clinical applications. DTI is mainly designed to study the diffusion of water molecules within biological tissues and to assess the microstructural organization of white matter tracts.20-22 In contrast, DIR focuses on suppressing specific tissue contrasts, such as cerebrospinal fluid (CSF) or white matter, to enhance the visibility of certain pathological features, such as lesions in neurological conditions.
DTI uses EPI sequences, while DIR employs GRE or FSE sequences. Both techniques have a field of view (FOV) in the range of 220–240 mm. Their slice thickness differs, with DTI offering thinner slices of 2–3 mm for improved spatial resolution and DIR using thicker slices of 3–5 mm for better signal-to-noise (S/N) ratio. Similarly, the resolution matrix size of DTI is usually 128 × 128 or higher, whereas DIR is 256 × 256 or higher. As for acquisition parameters, DTI uses a longer TR of 3000–10,000 ms and a short TE of 60–100 ms to optimize diffusion sensitivity. DIR, depending on the subtype, uses a TR of 3000–6000 ms, with GRE sequences requiring shorter TE (20–30 ms) and FSE sequences using longer TE (80–100 ms). Unlike DIR, DTI incorporates diffusion-specific parameters such as b-values (commonly b = 0 and b = 1000 s/mm2) and diffusion directions (minimum of six non-collinear directions, up to 64 for higher angular resolution), which are crucial for tensor calculations and tractography.
Both DTI and DIR permit axial, coronal, or sagittal slice orientation but differ in optional parameters and special considerations. DTI employs advanced gradient strength, partial Fourier factor, and parallel imaging to reduce artifacts, along with motion correction and eddy current correction for accurate tensor analysis. DIR focuses on fat saturation, motion correction, and the adjustment of TI to suppress specific tissue signals, with image fusion often used for enhanced diagnostic capabilities. It excels in detecting subtle microstructural changes, aiding in the study of neurological conditions such as MS, stroke, and traumatic brain injury.23 Additionally, DTI provides quantitative measures such as FA and MD, enabling the quantification of tissue integrity and pathology.24 Its applications extend to research, where it is widely utilized in neuroscience studies focusing on brain development, aging, and various neurological disorders. DTI’s strengths lie in its ability to offer comprehensive insights into white matter integrity and pathology, making it a valuable tool in both clinical and research settings. We analyzed data from reliable databases like Scopus, PubMed, SCI and Web of Science to draw conclusions in Table 2.
Review | Practical implications | Methods | Outcomes | Summary |
---|---|---|---|---|
Study on DTI role in brain tumor evaluation. Focus on white matter tracts involvement and tumor differentiation.16 |
DTI useful in the evaluation of brain tumors, including differentiation of low- versus high-grade gliomas. DTI helpful in differentiating gliomas from metastasis. |
This was a prospective study on patients with brain neoplasm conducted in the Department of Radiodiagnosis, Prathima Institute of Medical Sciences, Karimnagar. Using inclusion criteria, n=30 patients referred to Neurosurgery |
The most common were high-grade gliomas, 43.3%. DTI is useful in the evaluation of cerebral neoplasms and differentiation between tumors. |
DTI is useful in evaluating brain tumors since it differentiates between low-grade and high-grade gliomas. DTI more effective than MRI in cerebral neoplasm evaluation. |
Integrating DTI for microstructural changes and DIR for cortical lesion detection enhances brain white matter disease assessment, leveraging the strengths of each imaging modality.14 | AI-generated images reliable for the detection of lesions from multiple sclerosis. AI can be of use in diagnosis and follow-up on diseases. |
It generates AI-based DIR and PSIR images using generative adversarial networks. Randomized blinded scoring for lesion detection by seven experienced readers. MRI data of 202 patients with multiple sclerosis, mean age 46 years ±11 [SD], 127 women, retrospectively collected from seven centers from February 2020 to January 2021. |
AI-generated images detected more lesions than MRI-acquired images. Good reliability and interpretative agreement in a multicentre setting. |
AI-generated DIR and PSIR images compared well with the MRI-acquired images. AI-generated images can thus be considered reliable in a multicentre setting. |
Study compares DTI metrics in cerebral vascular lesions to NAWM. Associations between FA in NAWM and CVD risk factors.17 |
DTI metrics can serve to define the severity of brain vascular anomalies. FA in NAWM was linked to hypertension and disability evaluation. |
Comparison of DTI metrics in various cerebral vascular lesions. Segmentation of 10 different tissue/lesion classes for analysis. 152 individuals with CVD participating in the Ontario Neurodegenerative Disease Research Initiative. |
DTI metrics differed significantly in vascular lesions compared to healthy tissue. FA in NAWM inversely related to hypertension and disability severity. |
DTI metrics differ across vascular lesions and healthy tissue types. FA in NAWM inversely related to hypertension and disability. |
DTI in MS optic radiation reveals tissue-specific diffusivity patterns. Lesional and non-lesional fibers show distinct diffusivity alterations.18 |
DTI may potentially be a surrogate marker for monitoring MS progression and therapeutic efficiency. Lesional and non-lesional fibers show different diffusivity patterns in MS. |
Tract-specific DTI analysis. Segmentation of optic radiation into different compartments. In the optic radiation (OR) of 50 relapsing-remitting MS patients, several compartments were segmented: T2 lesions, the proximal and the distal portions of the fibers transacted by a lesion, and the fibers with no discernable pathology throughout the entire length of the OR. | Increased radial diffusivity in lesional fibers is related to myelin loss. Axial diffusivity elevation in the distal lesional fibers due to degeneration. | Increased radial diffusivity in lesional fibers is related to myelin loss. Axial diffusivity elevation in the distal lesional fibers due to degeneration. |
Study on microstructural lesions in Alzheimer’s spectrum using diffusion tensor imaging. Investigated diagnostic accuracy in different stages of Alzheimer’s disease.19 |
DTI parameters useful for MCI diagnosis, not for preclinical AD. Multicenter DTI shows a very high accuracy in diagnosing MCI cases. |
Diagnostic accuracy tested within a cross-validation framework. They examined the integrity of fiber tracts in limbic and association fiber tracts in MCI and AD dementia versus controls in a tract-based way. In a prospective cohort of the DELCODE study, comprising 271 subjects including 93 healthy controls and 98 subjective cognitive decline, 45 MCI, and 35 AD dementia cases, we found reductions of fiber tract integrity in limbic and association fiber tracts | DTI Parameters Useful for MCI Diagnosis but Not for Preclinical AD. High diagnostic accuracy for Aβ positive MCI cases. |
In particular, DTI helps in the diagnosis of MCI and Aβ positive cases. Automated analysis not useful for preclinical AD identification. |
in MCI and AD dementia compared to controls in a tract-based analysis (p < 0.05, family wise error corrected). | ||||
ENIGMA-DTI studies white matter deficits in psychiatric disorders globally. Identifies patterns, reproducibility, and individual vulnerability metrics.51 |
Identification of White Matter Deficits for Individualized Metrics in Psychiatric Disorders. It places a focus on cross-diagnostic features and individual vulnerability in neuropsychiatric research. |
Collaborative analysis by the ENIGMA workgroup. Standardized neuroimaging methods in multisite research. This delivered the largest reports of white matter deficit patterns in schizophrenia spectrum disorder, bipolar disorder, major depressive disorder, obsessive-compulsive disorder, post-traumatic stress disorder, traumatic brain injury, and 22 q11 deletion syndrome. |
Identified white-matter deficit patterns in different psychiatric disorders. Introduced regional vulnerability index for personalization of vulnerability metrics. |
The paper covers white matter deficits in major psychiatric disorders. It proposes the use of a metric called the regional vulnerability index in cross-diagnostic neuropsychiatric research. |
DTI analyzes cerebral white matter in neurodegenerative diseases. Investigates differences in WM patterns using various DTI analyses.52 |
Define distinct white matter patterns in neurodegenerative diseases. The performance of a longitudinal individual subject analysis charts disease progression. | Voxel wise comparison of regional diffusion direction-based metrics. Fiber tracking accompanied by tract-wise fractional anisotropy statistics. | Defines distinct WM Patho anatomy of brain diseases. It combines whole-brain-based and tract-based DTI analyses. | DTI defines distinct WM Patho anatomy in various brain diseases. It offers DTI analysis techniques for quantitative comparison between groups. |
DKI combines diffusivity and kurtosis for Alzheimer’s detection. Study enhances DKI capacity in pathologic white matter alterations53 |
Improved Detection of Alzheimer’s: A Combined Diffusivity and Kurtosis-Based Approach in DKI. It had an accuracy of 96.23% with the combined method in the detection of Alzheimer’s. |
Support vector machine-based approach. It is a combination of diffusivity and kurtosis within DKI. On combination, 53 subjects—27 with Alzheimer’s—achieved accuracy performances of 96.23%. |
It can detect Alzheimer’s disease with 96.23% accuracy. This will bring more accurate diffusivity and complementary kurtosis, thus contributing to performance. | The combination of diffusivity and kurtosis increases the accuracy in Alzheimer’s detection. High performance is due to more precise diffusivity and complementary kurtosis. |
Multicenter study on Alzheimer’s using machine learning for DTI data. Classification of white matter degeneration in Alzheimer’s patients.53 |
Robust automated detection of white matter degeneration in Alzheimer’s. Machine learning classification supports the applicability of multicentre DTI data. |
Classification of machine learning with SVM and NB, as well as the principal component analysis for reducing the variance of multicentre data, were carried out. They included a sample of 137 patients diagnosed with clinically probable AD (MMSE 20.6±5.3) and 143 healthy elderly controls, all scanned in nine different scanners. | SVM accuracy: 80% for FA and 83% for MD. NB accuracy: it is from 68% to 75% in pooled cross-validation. |
For FA, SVM achieved an accuracy of 80%, while MD stood at 83%. Y: 68-75% in pooled cross-validation. |
DTI: Diffusion tensor imaging, NAWM: Non-lesional white matter, CVD: Cardio vascular diseases, AI: Artificial intelligences, PSIR: Phase-sensitive inversion recovery, SD: Standard deviation, FA: Fractional anisotropy, MS: Multiple sclerosis, AD: Alzheimer’s disease, MCI: Mild cognitive impairment, DKI: Difussion kurtosis imaging, WM: White matter, SVM: Support vector machine, MD: Mean diffusivity, NB: Naive bayes, ENIGMA-DTI: Enhancing neuroimaging genetics through meta-analysis consortium, DKI: Difussion kurtosis imaging, Y: Variances, DELCODE: Developmental and environmental life course outcomes of diet and exercise, DIR: Deformation-insensitive registration, MRI: Magnetic resonance imaging, MMSE: Mini-mental state examination
DTI exhibits limitations such as limited spatial resolution, especially when compared to structural MRI.25 Moreover, DTI is highly sensitive to motion artifacts, which is particularly problematic in pediatric and patient populations, potentially compromising image quality and analysis accuracy.24 Another weakness lies in DTI’s struggle in accurately resolving crossing fibers, especially in areas where white matter fibers intersect, potentially leading to inaccuracies in tractography results.26 DTI’s assumption of a single fiber orientation within each voxel poses challenges in capturing complex fiber architectures, like regions with crossing, kissing, or fanning fibers, limiting its ability to represent intricate brain structures.27
Diffusion-weighted imaging with resting-state fMRI (DIR) is a powerful technique that merges structural insights from DWI with functional connectivity data from resting-state fMRI, offering a holistic view of brain structure-function relationships.28,29 By combining structural connectivity mapping with functional correlation analysis, DIR enables the exploration of how brain regions interact both structurally and functionally during rest.30 Moreover, DIR can pinpoint functional networks and investigate how they are modulated by underlying structural connections, facilitating the examination of brain networks involved in diverse cognitive processes.31 With promising clinical applications, DIR holds the potential to unravel changes in structural and functional connectivity patterns associated with neurological and psychiatric disorders, showcasing its significance in advancing the understanding of brain disorders.32
Complex data integration is a significant weakness when combining DIR data, requiring intricate processing techniques.33 In addition, sensitivity to motion artifacts, common in DTI and DIR, necessitates meticulous correction strategies during data processing.34 The interpretation of DIR data poses challenges, demanding expertise in both diffusion imaging and functional MRI, which can be a barrier for researchers and clinicians unfamiliar with both modalities.35 Moreover, DIR faces limitations in spatial and temporal resolution inherited from diffusion-weighted imaging and resting-state fMRI, potentially hindering the resolution of fine anatomical details and rapid changes in brain connectivity.36
Diffusion Tensor Imaging (DTI) and Diffusion-Weighted Imaging with Resting-state fMRI (DIR) complement each other
DTI and diffusion-weighted imaging with resting-state fMRI complement each other in understanding brain connectivity.37,38 DIR integrates structural data from DTI with functional connectivity from resting-state fMRI, revealing how brain structure influences function, while DTI provides detailed maps of white matter tracts, aiding in understanding the physical pathways underlying functional connections.39 DIR identifies functional networks and integrates them with structural connectivity data, while DTI visualizes white matter tracts and quantifies microstructural properties, providing the structural basis of functional networks. Both techniques offer a comprehensive approach to brain mapping, with DIR simultaneously assessing structural and functional connectivity and DTI widely used in clinical research to investigate neurological conditions and disease progression. In addition, DIR holds promise for studying neurological and psychiatric disorders, while DTI is instrumental in understanding brain connectivity and the structural basis of cognition and behavior.
Potential for combined use of DIR and DTI in clinical practice
Combining diffusion-weighted imaging with resting-state fMRI (DIR) and diffusion tensor imaging (DTI) holds great potential for clinical practice, offering a comprehensive approach to understanding brain structure and function. Here is how the combined use of DIR and DTI can benefit clinical practice:
-
1.
Early detection and diagnosis: DIR, utilizing resting-state fMRI, can detect functional connectivity alterations preceding structural changes.40 On the other hand, DTI excels in identifying microstructural changes in white matter tracts before they manifest on conventional MRI scans, facilitating early detection of conditionslike MS, Alzheimer’s disease, and Parkinson’s disease.41 By leveraging the complementary strengths of DIR and DTI, healthcare professionals can potentially enhance their ability to diagnose these disorders at earlier stages, enabling timely interventions and improved patient outcomes.42
-
2.
Treatment planning and monitoring: DTI aids in assessing white matter damage, particularly in conditions such as traumatic brain injury and stroke, enabling the identification of damage extent, guiding rehabilitation strategies, and monitoring treatment response. On the other hand, DTI can provide critical information for treatment planning by predicting therapy response and identifying intervention targets, such as deep brain stimulation, through the assessment of structural and functional connectivity.43,44 The integration of DTI and DTT can enhance patient care by offering insights into neural connectivity, structural changes, and potential prognostic indicators, ultimately improving treatment outcomes and patient recovery.
-
3.
Understanding disease mechanisms: The integration of DIR and DTI enables a comprehensive exploration of structural and functional alterations in neurological and psychiatric disorders, shedding light on disease mechanisms and facilitating the development of precise treatment strategies.45,46 DTI, on the other hand, plays a crucial role in identifying distinct white matter damage patterns linked to various conditions, aiding in the comprehension of how these alterations correspond to clinical manifestations and disease advancement.47,48 By combining the capabilities of DIR and DTI, clinicians can gain a deeper understanding of the intricate interplay between structural changes, functional disruptions, and disease progression, ultimately paving the way for more targeted and effective therapeutic interventions.
-
4.
Multimodal imaging biomarkers: Combining DTI with diffeomorphic anatomical registration through exponentiated Lie algebra (DARTEL) enables the creation of multimodal imaging biomarkers that encompass both structural and functional characteristics of brain connectivity.49 These advanced biomarkers offer significant potential for enhancing diagnostic precision, tracking disease advancement, and evaluating the effectiveness of treatments in clinical settings. By integrating DTI and DARTEL, researchers can obtain a comprehensive understanding of neuropathological signatures in conditions such as amyotrophic lateral sclerosis (ALS) and MS, facilitating individualized patient stratification and improving the accuracy of diagnostic classifications.50 This multimodal approach is promising for revolutionizing neuroimaging assessments and developing novel biomarkers for a more detailed evaluation of brain disorders.
DISCUSSION
The utilization of MRI sequences such as DIR and DTI is pivotal in diagnosing brain white matter diseases. DIR sequences excel in enhancing the visibility of cortical lesions in conditions such as MS, outperforming traditional sequences like FLAIR. DTI provides valuable insights into white matter integrity by measuring water molecule diffusion, aiding in the assessment of diseases such as MS. These techniques offer complementary benefits, with DIR pinpointing cortical lesions effectively while DTI unveils microstructural changes in white matter tracts. The importance of white matter integrity lies in its role as a network connecting various regions of the brain. Myelinated axons, oligodendrocytes, and glial cells compose white matter, which is crucial for efficient signal transmission. The myelin sheaths enveloping axons play a pivotal role in signal conduction and neural communication. Understanding these structural components and their interactions is vital for comprehending brain function and pathology. The review paper discusses the limitations of DTI in diagnosing conditions like cerebello-pontine angle (CPA) tumors.54 It emphasizes the importance of preserving the facial nerve (FN) during CPA tumor surgery and highlights the suboptimal results of FN tracking with DTI. On the other hand, clinical decision support systems (CDSS) play a crucial role in impacting clinical decision-making.55 They utilize computational models such as support vector machine (SVM) and Decision Tree to aid in diagnosing heart and stroke diseases. In addition, the review on multimorbidity emphasizes the need for more evidence on how different conditions interact to improve patient safety.56 Integrating the strengths of DTI for precise imaging and CDSS for decision support can enhance patient care by providing accurate diagnoses and treatment planning in complex scenarios like multimorbidity or conditions requiring detailed neural imaging.
Methodologically, both DIR and DTI demonstrate strength in diagnosing white matter diseases. DIR has shown efficacy in identifying cortical lesions, especially in MS patients, while DTI provides detailed information on microstructural changes associated with various conditions, including aging, Alzheimer’s disease, and cerebrovascular disease. Integration of DTI and DIR methodologies can offer a comprehensive assessment of brain white matter diseases, leveraging their strengths for a more thorough evaluation. Recent advancements in neuroimaging, including machine learning techniques, have further enhanced the diagnostic capabilities of DIR and DTI. Artificial intelligence (AI)-generated images have proven reliable in lesion detection in MS patients, offering the potential for diagnostic and disease monitoring purposes. In addition, DTI studies have utilized machine learning algorithms to identify white matter deficits in psychiatric disorders and neurodegenerative diseases.
CONCLUSION
DIR and DTI are invaluable tools in the diagnosis and evaluation of brain white matter diseases. Their complementary benefits, when integrated with advanced imaging techniques and machine learning algorithms, offer a comprehensive approach to understanding and managing these conditions. The integration of DWI with DTI sequences in medical imaging holds significant importance in enhancing diagnostic capabilities. These sequences provide valuable insights into tissue microstructure and connectivity, aiding in the detection and characterization of various pathologies. By combining these imaging modalities with advanced techniques and machine learning algorithms, such as deep learning, the potential benefits are substantial. Advanced algorithms can leverage the rich information provided by DWI and DTI to improve accuracy in disease diagnosis, treatment planning, and monitoring. The synergy between these imaging modalities and machine learning approaches offers a promising avenue for more precise and personalized healthcare interventions, ultimately leading to enhanced patient care and outcomes. Future research directions in imaging for white matter disease detection involve the integration of advanced techniques like b-tensor encoding schemes in diffusion-weighted MRI with machine learning. In addition, the development of automated segmentation methods utilizing intensity standardization, texture feature extraction, and machine learning algorithms can enhance the detection of subtle white matter lesions. Exploring the role of white matter inflammation, microglial activation, and astrocytosis in neurodegenerative conditions such as Alzheimer’s disease can provide insights into early biomarkers for cognitive decline. Future studies could focus on refining imaging modalities to detect microstructural changes indicative of disease progression, leveraging machine learning for accurate segmentation, and investigating the relationship between white matter abnormalities and cognitive outcomes for improved diagnostic and therapeutic strategies.
Author contribution
DK, SG: Study conception and design; DK: Data collection; RR: Analysis; DK, SG, SD,: Draft manuscript preparation. All authors reviewed the results and approved the final version of the manuscript.
Ethical approval
Institutional Review Board approval is not required.
Declaration of patient consent
Patient’s consent is not required as there are no patients in this study.
Financial support and sponsorship
Nil.
Conflicts of interest
There are no conflicts of interest.
Use of artificial intelligence (AI)-assisted technology for manuscript preparation
The authors confirm that there was no use of artificial intelligence (AI)-assisted technology for assisting in the writing or editing of the manuscript and no images were manipulated using AI.
REFERENCES
- Synthetic double inversion recovery imaging in brain MRI: Quantitative evaluation and feasibility of synthetic MRI and a comparison with conventional double inversion recovery and fluid-attenuated inversion recovery sequences. BMC Med Imaging. 2022;22:183.
- [CrossRef] [PubMed] [PubMed Central] [Google Scholar]
- Comparative between double inversion recovery and fluid-attenuated inversion recovery sequences for detection of brain multiple sclerosis. Clin Cancer Investig J. 2022;11:41-4.
- [CrossRef] [Google Scholar]
- Comparative study between double inversion recovery (DIR) and fluid-attenuated inversion recovery (FLAIR) MRI sequences for detection of cerebral lesions in multiple sclerosis. Egypt J Radiol Nucl Med. 2020;51:188.
- [CrossRef] [Google Scholar]
- Human brain white matter function analysis based on functional gradient. In: 2022 4th International Conference on Frontiers Technology of Information and Computer (ICFTIC). Qingdao, China: IEEE; 2022. p. :1061-1065. Available from https://doi.org/10.1109/ICFTIC57696.2022.10075268
- [Google Scholar]
- White matter: Basic principles of axonal organization and function. In: White matter injury in stroke and CNS disease. New York, NY: Springer New York; 2014. p. :3-38.
- [Google Scholar]
- Incorporating susceptibility effects into the standard model of diffusion in white matter. In: ISMRM workshop on diffusion MRI. 2022.
- [Google Scholar]
- Differentiation of white matter histopathology using b-tensor encoding and machine learning. PLoS One. 2023;18:e0282549.
- [CrossRef] [PubMed] [PubMed Central] [Google Scholar]
- Disc-diff: Disentangled conditional diffusion model for multi-contrast mri super-resolution. In: International Conference on Medical Image Computing and Computer-Assisted Intervention. Cham: Springer Nature Switzerland; 2023. p. :387.
- [Google Scholar]
- Spatial alignment of structural images on single-shot and readout-segmented (RESOLVE) diffusion images in the optic nerve for tractography template generation. Authorea Preprints 2023 Available from: http://dx.doi.org/10.22541/au.167889616.62406935/v1
- [Google Scholar]
- Multiple sclerosis versus cerebral small vessel disease in MRI: A practical approach using qualitative and quantitative signal intensity differences in white matter lesions. Acta Radiol. 2024;65:106-14.
- [CrossRef] [PubMed] [Google Scholar]
- Diffusion tensor imaging (DTI) studies of cerebral white matter integrity in normal to moderate cardiovascular risk patients. Neurol Asia. 2023;28:131-9.
- [CrossRef] [Google Scholar]
- Comparison of diffusion tensor imaging metrics in normal-appearing white matter to cerebrovascular lesions and correlation with cerebrovascular disease risk factors and severity. Int J Biomed Imaging. 2022;2022:5860364.
- [CrossRef] [PubMed] [PubMed Central] [Google Scholar]
- Modeling the properties of white matter tracts using diffusion tensor imaging to characterize patterns of injury in aging and neurodegenerative disease. Front Aging Neurosci. 2022;14:787516.
- [CrossRef] [PubMed] [PubMed Central] [Google Scholar]
- Diffusion-weighted magnetic resonance imaging in acute stroke. Stroke. 1998;29:1783-90.
- [CrossRef] [PubMed] [Google Scholar]
- Decoding diffusivity in multiple sclerosis: Analysis of optic radiation lesional and non-lesional white matter. PLoS One. 2015;10:e0122114.
- [CrossRef] [PubMed] [PubMed Central] [Google Scholar]
- Multicenter tract-based analysis of microstructural lesions within the alzheimer’s disease spectrum: Association with amyloid pathology and diagnostic usefulness. J Alzheimers Dis. 2019;72:455-65.
- [CrossRef] [PubMed] [PubMed Central] [Google Scholar]
- White matter anatomy of human brain using DTI based atlas: A pictoral review at 3T;2023; Available from: https://doi.org/10.21203/rs.3.rs-2903660/v1
- Clinical applications of brain diffusion-tensor imaging and fiber tractography. The Scientific Journal of Al-Azhar Medical Faculty, Girls. 2022;6:57-63.
- [CrossRef] [Google Scholar]
- Modeling the properties of white matter tracts using diffusion tensor imaging to characterize patterns of injury in aging and neurodegenerative disease. Front Aging Neurosci.. 2022;14
- [CrossRef] [Google Scholar]
- Diffusion tensor imaging. In: Neuroimaging techniques in clinical practice. Cham: Springer International Publishing; 2020. p. :203-13.
- [Google Scholar]
- Diffusion tensor imaging color-coded maps: An alternative to tractography. Stereotact Funct Neurosurg. 2021;99:295-304.
- [CrossRef] [PubMed] [Google Scholar]
- Improvement in white matter tract reconstruction with constrained spherical deconvolution and track density mapping in low angular resolution data: A pediatric study and literature review. Front Pediatr. 2017;5:182.
- [CrossRef] [PubMed] [PubMed Central] [Google Scholar]
- Strengths and limitations of tractography methods to identify the optic radiation for epilepsy surgery. Quant Imaging Med Surg. 2015;5:288-99.
- [CrossRef] [PubMed] [PubMed Central] [Google Scholar]
- Inherent limitations of tractography for accurate connectivity maps. Neurosurgery. 2015;76:N11-2.
- [CrossRef] [PubMed] [Google Scholar]
- The usefulness and limitations of diffusion tensor imaging – A review study. Eur J Transl Clin Med. 2020;2:43-51.
- [Google Scholar]
- Attention-diffusion-bilinear neural network for brain network analysis. IEEE Trans Med Imaging. 2020;39:2541-52.
- [CrossRef] [PubMed] [Google Scholar]
- Voxel-wise brain graphs from diffusion MRI: Intrinsic eigenspace dimensionality and application to functional MRI. IEEE Open J Eng Med Biol. 2023;6:158-67.
- [CrossRef] [PubMed] [PubMed Central] [Google Scholar]
- Diffusion MRI in peripheral nerves: Optimized b values and the role of non-gaussian diffusion. Radiology. 2022;302:153-61.
- [CrossRef] [PubMed] [Google Scholar]
- Diffusion tensor MRI structural connectivity and pet amyloid burden in preclinical autosomal dominant Alzheimer disease: The DIAN cohort. Radiology. 2022;302:143-50.
- [CrossRef] [PubMed] [PubMed Central] [Google Scholar]
- Optimized diffusion imaging for brain structural connectome analysis. IEEE Trans Med Imaging. 2022;41:2118-29.
- [CrossRef] [PubMed] [PubMed Central] [Google Scholar]
- Application of sophisticated models to conventional diffusion-weighted MRI data 2018.
- Using light and X-ray scattering to untangle complex neuronal orientations and validate diffusion MRI. Elife. 2023;12:e84024.
- [CrossRef] [PubMed] [PubMed Central] [Google Scholar]
- Validating the accuracy of multispectral metal artifact suppressed diffusion-weighted imaging. Med Phys. 2022;49:6538-46.
- [CrossRef] [PubMed] [PubMed Central] [Google Scholar]
- What does diffusion tensor imaging (DTI) tell us about cognitive networks in temporal lobe epilepsy? Quant Imaging Med Surg. 2015;5:247-63.
- [CrossRef] [PubMed] [PubMed Central] [Google Scholar]
- Diffusion tensor imaging. Surgery of spinal cord tumors based on anatomy: An approach based on anatomic compartmentalization. 2021:147-51.
- [Google Scholar]
- Impact of diffusion tensor field smoothing with Log-Cholesky metric on noise reduction. Frontiers in Biomedical Technologies. 2024.
- New insights into the pathology of white matter tracts in cerebral palsy from diffusion magnetic resonance imaging: a systematic review. Developmental Medicine & Child Neurology. 2012;54:684-96.
- [CrossRef] [PubMed] [Google Scholar]
- DTI in psychiatry. In: Diffusion tensor imaging. New York, NY: Springer New York; 2016. p. :359-72.
- [Google Scholar]
- Application of magnetic resonance DTI technique in evaluating the effect of postoperative exercise rehabilitation. J Healthc Eng. 2022;2022:2385699.
- [CrossRef] [PubMed] [PubMed Central] [Google Scholar]
- What can DTI add in acute ischemic stroke patients? Egypt J Radiol Nucl Med. 2019;50:67.
- [CrossRef] [Google Scholar]
- Histopathological correlation of diffusion basis spectrum imaging metrics of a biopsy-proven inflammatory demyelinating brain lesion: A brief report. Mult Scler. 2019;25:1937-41.
- [CrossRef] [PubMed] [PubMed Central] [Google Scholar]
- The role of DTI in early detection of cervical spondylotic myelopathy: a preliminary study with 3-T MRI. Neuroradiology. 2011;53:609-16.
- [CrossRef] [PubMed] [Google Scholar]
- White matter microstructural changes using ultra-strong diffusion gradient MRI in adult-onset idiopathic focal cervical dystonia. Neurology. 2024;103:e209695.
- [CrossRef] [PubMed] [PubMed Central] [Google Scholar]
- Applying dimensional psychopathology: Transdiagnostic prediction of executive cognition using brain connectivity and inflammatory biomarkers. Psychol Med. 2023;53:3557-67.
- [CrossRef] [PubMed] [Google Scholar]
- Evaluation of MRI and cannabinoid type 1 receptor PET templates constructed using DARTEL for spatial normalization of rat brains. Med Phys. 2015;42:6875-84.
- [CrossRef] [PubMed] [Google Scholar]
- The impact of edema and fiber crossing on diffusion MRI metrics assessed in an ex vivo nerve phantom: Multi-tensor model vs. diffusion orientation distribution function. NMR Biomed. 2021;34:e4414.
- [CrossRef] [PubMed] [PubMed Central] [Google Scholar]
- Are current machine learning applications comparable to radiologist classification of degenerate and herniated discs and Modic change? A systematic review and meta-analysis. Eur Spine J. 2023;32:3764-3787.
- [CrossRef] [PubMed] [PubMed Central] [Google Scholar]
- Diffusion tensor imaging to detect early signs of neurodegenerative disease. In: 2024 15th International conference on computing communication and networking technologies (ICCCNT). Kamand, India: IEEE; 2024. p. :1-6.
- [Google Scholar]
- Automated detection of pathologic white matter alterations in Alzheimer’s disease using combined diffusivity and kurtosis method. Psychiatry Res Neuroimaging. 2017;264:35-45.
- [CrossRef] [PubMed] [Google Scholar]
- AI4AD: Artificial intelligence analysis for Alzheimer’s disease classification based on a multisite DTI database. Brain Disorders. 2021;1:100005.
- [Google Scholar]
- Commentary on: The usefulness and limitations of diffusion tensor imaging – A review study. Eur J Transl Clin Med. 2020;2:85-6.
- [Google Scholar]
- Effects of computer-based clinical decision support systems on physician performance and patient outcomes: a systematic review. Jama. 1998;280:1339-46.
- [CrossRef] [PubMed] [Google Scholar]
- ENIGMA-DTI: Translating reproducible white matter deficits into personalized vulnerability metrics in cross-diagnostic psychiatric research. Hum Brain Mapp. 2022;43:194-206.
- [CrossRef] [PubMed] [PubMed Central] [Google Scholar]