Translate this page into:
Evaluating the role of artificial intelligence in automated image analysis for x-ray radiography
Corresponding author: Dheeraj Kumar, Department of Medical Radiology and Imaging Technology, School of Health Sciences, Chhatrapati Shahu Ji Maharaj University, Kanpur, India. dheeraj199494@gmail.com
-
Received: ,
Accepted: ,
How to cite this article: Kumar D, Diwakar SK, Gupta S. Evaluating the role of artificial intelligence in automated image analysis for x-ray radiography. Future Health. 2024;2:52–57. doi: 10.25259/FH_14_2024
Abstract
This article intends to assess how artificial intelligence (AI) affects the automation of X-ray radiography image processing. The field of medical imaging has seen considerable potential in the use of AI algorithms to improve diagnostic precision, streamline procedures, and streamline workflow. The paper explores how AI is currently being used to automate image processing for X-ray radiography, outlining its possible benefits, difficulties, and hopes for the future. According to the results, AI has the potential to revolutionize radiography by helping radiologists evaluate images, locate anomalies, and do quantitative analysis. This discovery may result in important improvements in healthcare. To determine the clinical value and safety of AI-driven solutions, it is necessary to carry out more research and validation. In conclusion, this analysis highlights AI’s critical role in automating image processing for X-ray radiography and demonstrates how it has the potential to completely transform the industry. To guarantee the dependability and efficacy of AI-based techniques in clinical practice, however, continual research and validation are crucial.
Keywords
Artificial Intelligence
Automated Image Analysis
X-ray Radiography
Diagnostic Accuracy
Efficiency
Workflow
INTRODUCTION
X-ray radiography is a popular medical imaging modality for identifying a wide range of diseases and conditions. Radiologists are essential for analyzing X-ray pictures in order to discover abnormalities and make correct diagnoses; they also help radiographers with new technology and give suitable treatment strategies.1 Manual picture analysis, on the other hand, is time-consuming, subjective, and prone to human mistakes. There has been an increasing interest in using artificial intelligence (AI) approaches to automate image processing in radiography in recent years.2
AI’s current position in automated image analysis in radiography
The use of AI algorithms in automated image processing for X-ray radiography has piqued the interest of the medical imaging community [Figure 1]. Deep learning and machine learning have shown promising outcomes in a variety of responsibilities, including picture segmentation, abnormality detection, and quantitative analysis. Several researches have shown that AI has the potential to improve diagnostic accuracy and efficiency in radiography.3
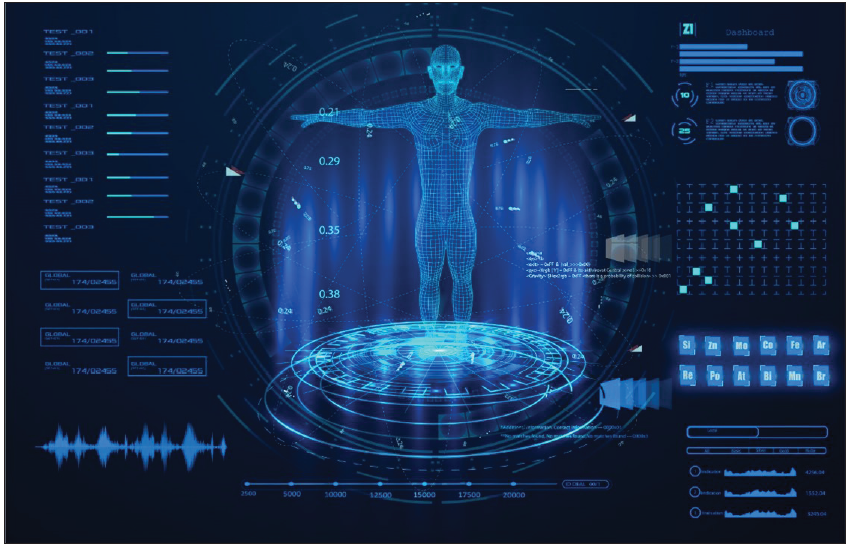
- This is AI in radiography picture.
Researchers, for example, have created AI-based algorithms that can detect and localize anomalies in X-ray images, such as lung nodules, fractures, and tumors. Convolutional neural networks (CNNs) are used in these algorithms to learn characteristics from big datasets and find patterns linked with specific diseases or disorders [Figure 2a-2g]. AI algorithms have been found in studies to perform as well as or better than human radiologists in detecting abnormalities in X-ray pictures.4,5
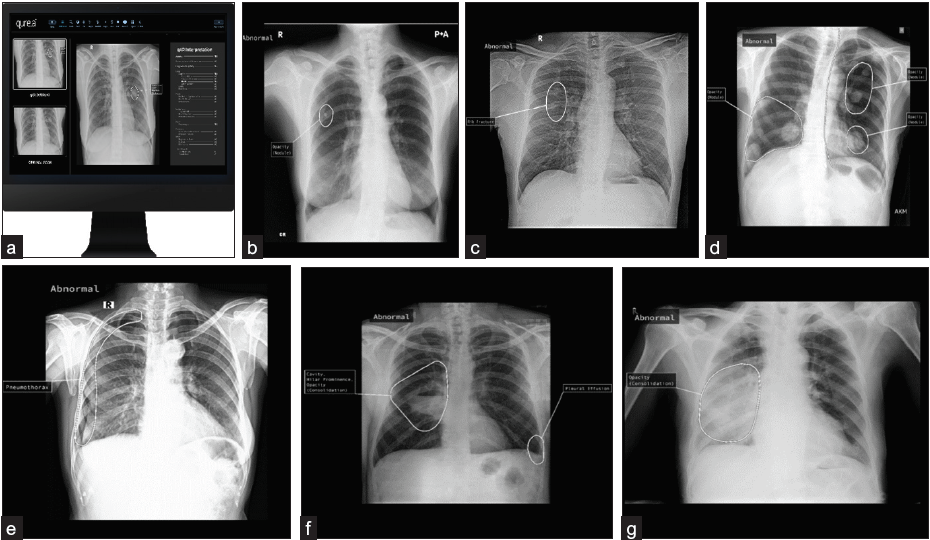
- The AI in radiography images automatic pathology prediction suggestions https://qure.ai/.
-
(a)
The 1st image might show a software interface designed for automatic pathology detection. It could feature a graphical user interface (GUI) with various elements such as buttons, dropdown menus, or input fields. The main area of the interface might display medical images such as X-rays radiography.
-
(b)
image depicts automated detection of a nodule with opacity in the right side of the chest PA view.
-
(c)
abnormality in the chest AP view is detected by the software, highlighting a possible rib fracture.
-
(d)
software detects abnormalities in the chest PA view, highlighting multiple nodular opacities.
-
(e)
image, the software detects abnormalities in the chest PA view, highlighting pneumothorax on the right side.
-
(f)
The software highlights right-sided cavity nodular prominence (consolidation) in the chest PA view.
-
(g)
The software highlights right-sided opacity (consolidation) in the chest PA view.
These pictures are the use of Qure.ai6 a game-changing AI solution provider that is upending the radiology “status quo” by boosting imaging accuracy and health outcomes with the use of machine-supported tools. Deep learning technology is used by Qure.ai to provide automated interpretation of radiological tests such as X-rays. Furthermore, AI systems can help radiologists with quantitative analysis by quantifying the size, volume, or density of anatomical structures or lesions automatically. This can be useful for illness staging, therapy planning, and tracking treatment response. AI algorithms may also be utilized to improve pictures and reduce noise, enhancing the quality of X-ray images and assisting with visual interpretation.7
-
1.
Personalized Medicine: Personalized medicine is one of the most intriguing applications of AI in X-ray radiography. AI algorithms may be used to optimize many elements of radiography by utilizing patient-specific data, such as medical history, genetic information, and treatment outcomes. 8
-
2.
Integration with Electronic Health Records (EHRs): The future of X-ray radiography will be significantly improved by the seamless integration of AI systems with EHRs. This integration provides a number of advantages:9
-
3.
Hybrid Imaging: A more thorough and accurate assessment of a patient’s condition is promised by AI’s integration with other imaging modalities, such as computed tomography (CT) and magnetic resonance imaging (MRI).10 A full assessment of a patient’s anatomy and pathology is possible by combining AI-enhanced X-ray radiography with CT and MRI data. Diagnoses that are more thorough and accurate might result from this synergy.11
-
4.
AI-Enhanced Education: By providing both existing practitioners and aspiring radiologists with useful information, AI-driven solutions have the potential to transform radiology education:11
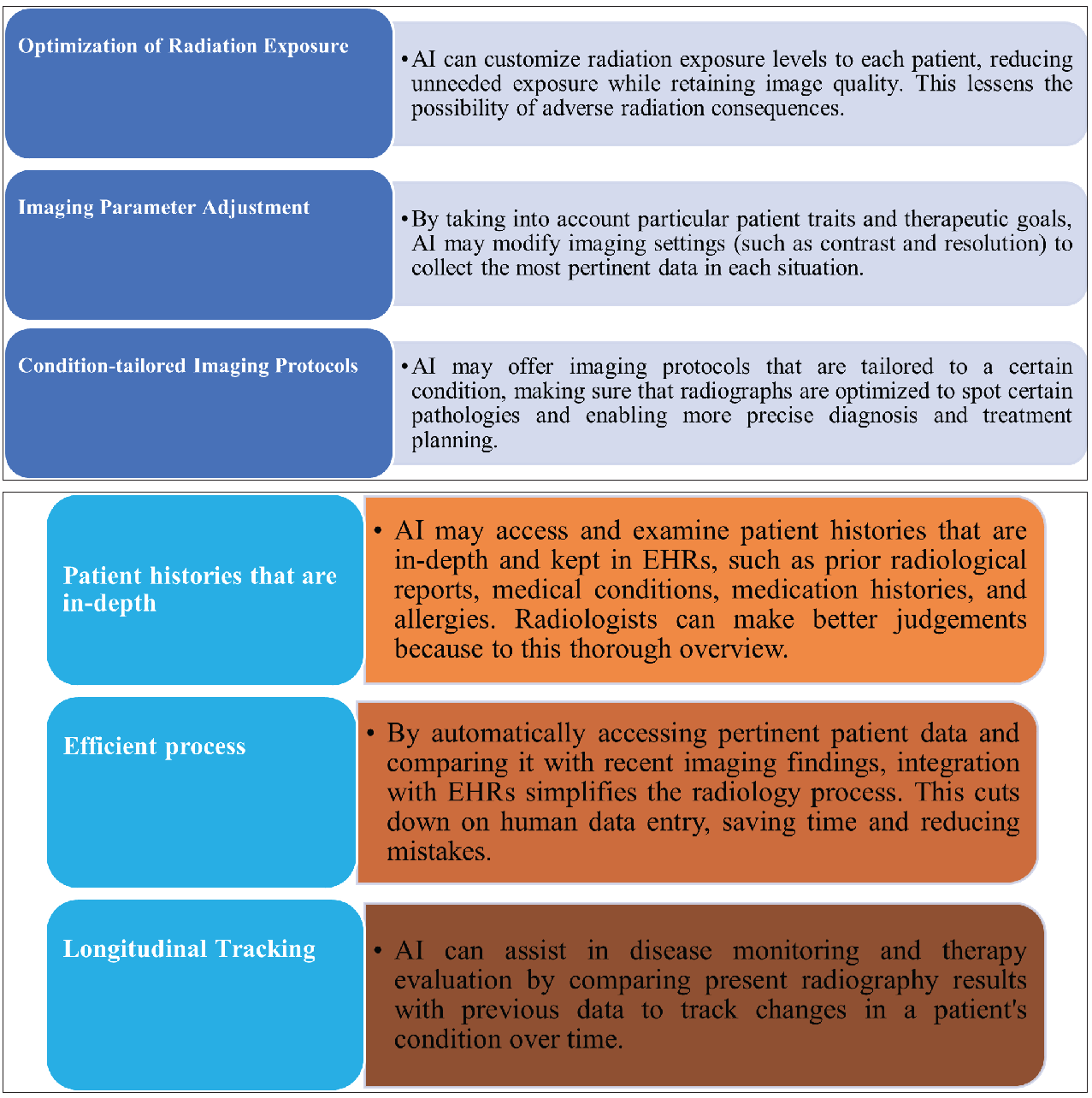
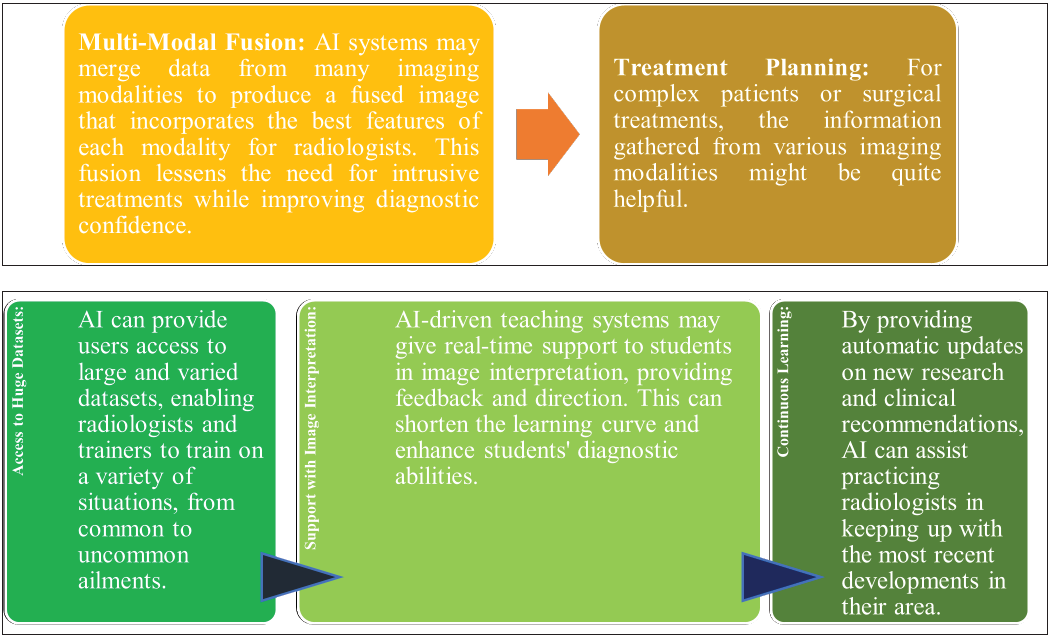
DISCUSSION
Despite the encouraging results, adopting AI-based automated image processing in radiography brings various obstacles and concerns. The necessity for big and diverse annotated datasets for training AI systems is one of the major problems.12 It is critical to have access to high-quality labeled data while developing accurate and resilient models. Obtaining annotated data, on the other hand, can be time-consuming and needs the skill of radiologists or health care professionals.13
Another difficulty is interpreting and explaining AI algorithms. Deep learning models, such as CNNs, are recognized for their black-box aspect, which makes understanding the underlying decision-making process challenging [Table 1].
This lack of interpretability raises issues about AI-based systems’ confidence and adoption in clinical practice. To solve this difficulty, efforts are being undertaken to construct explainable AI models and interpretability methodologies.14
In addition, incorporating AI algorithms into current radiology workflow and systems presents logistical and technological obstacles. AI-based system installation necessitates smooth connection with picture archiving and communication systems (PACS) and radiology information systems (RIS). To facilitate easy acceptance and incorporation into everyday practice, the compatibility, scalability, and performance of AI algorithms in real-world clinical contexts must be rigorously assessed.2 Furthermore, there are regulatory and ethical concerns with AI in radiography. Guidelines and criteria for the development, validation, and implementation of AI-based systems in healthcare must be established by regulatory agencies.13 To ensure patient safety and justice in healthcare delivery, ethical problems such as patient privacy, data security, and bias in AI systems must be addressed.15
Challenges and Concerns | Details |
---|---|
Data Challenges | Need for large, diverse annotated datasets for training AI. |
Obtaining high-quality labeled data is time-consuming. | |
Interpretability of AI Algorithms | Deep learning models like CNNs are often considered black-box. |
Lack of interpretability raises concerns about AI adoption. | |
Integration into Workflow | AI systems must seamlessly integrate with PACS and RIS. |
Compatibility, scalability, and performance are key considerations. | |
Regulatory and Ethical Concerns | Regulatory agencies need to establish guidelines for AI in healthcare. |
Ethical issues like patient privacy, data security, and bias must be addressed. |
AI: Artificial Intelligence; CNNs: Convolutional neural networks; PACS: Picture archiving and communication systems, RIS: Radiology information systems
CONCLUSION
Finally, the assessment of AI’s function in automated image processing for X-ray radiography highlights the possible benefits and obstacles of integrating AI-based systems in clinical practice. AI has demonstrated promising outcomes in radiography in terms of diagnosis accuracy, efficiency, and workflow. For an effective application, various problems must be solved, including the availability of annotated data, the interpretability of AI algorithms, integration into existing workflow, and legal and ethical constraints [Table 2].
Future research should concentrate on the creation of strong and interpretable AI algorithms, their validation in large multicenter trials, and the resolution of regulatory and ethical issues [Table 3]. To guarantee the safe and successful integration of AI in radiography, radiologists, computer scientists, and regulatory organizations must work together.
Key Findings | Details |
---|---|
Impact of AI on X-ray Radiography | AI can revolutionize radiography by improving diagnostic precision and efficiency. |
It streamlines workflow and aids in quantitative analysis. | |
Current Position of AI in Automated Image Analysis | Deep learning and machine learning show promise in tasks like image segmentation and anomaly detection. |
AI algorithms perform on par with or better than human radiologists in detecting abnormalities. | |
Potential Benefits of AI in Radiography | Personalized medicine, integration with EHRs, and hybrid imaging can enhance diagnosis and treatment. |
AI can transform radiology education. |
AI: Artificial intelligence; EHRs: Electronic health records
Future Directions | Details |
---|---|
Development of Strong and Interpretable AI Models | Research should focus on creating interpretable AI algorithms. |
Validation in large multicenter trials is necessary. | |
Resolution of Regulatory and Ethical Issues | Guidelines and criteria for AI-based systems in healthcare must be established. |
Address ethical concerns for patient safety and justice. | |
Collaboration between Radiologists and AI Experts | Radiologists, computer scientists, and regulatory organizations should work together. |
AI: Artificial intelligence
AI has the potential to revolutionize radiography by boosting radiologists’ talents and improving patient care with continuing breakthroughs in AI technology and thorough validation.
Ethical approval
Institutional Review Board approval is not required.
Declaration of patient consent
Patient’s consent is not required as patients identity is not disclosed or compromised.
Financial support and sponsorship
Nil
Conflicts of interest
There are no conflicts of interest
Use of artificial intelligence (AI)-assisted technology for manuscript preparation
The authors confirm that there was no use of artificial intelligence (AI)-assisted technology for assisting in the writing or editing of the manuscript and no images were manipulated using AI.
Bibliography
- Machine learning in x-ray diagnosis for oral health : a review of recent progress. 2023
- [Google Scholar]
- Assessment of radiology artificial intelligence software: a validation and evaluation framework. Can Assoc Radiol J 2022
- [Google Scholar]
- Multimodal image-text matching improves retrieval-based chest x-ray report generation. 2023:1-13.
- [Google Scholar]
- The current and future state of ai interpretation of medical images. N Engl J Med. 2023;388:1981-90.
- [CrossRef] [PubMed] [Google Scholar]
- Suboptimal chest radiography and artificial intelligence: the problem and the solution. Diagnostics (Basel). 2023;13:1-10.
- [Google Scholar]
- Artificial intelligence-reported chest X-ray findings of culture-confirmed pulmonary tuberculosis in people with and without diabetes. J Clin Tuberc Other Mycobact Dis. 2023;31:100365.
- [CrossRef] [PubMed] [PubMed Central] [Google Scholar]
- Evaluation of chest X-ray with automated interpretation algorithms for mass tuberculosis screening in prisons: A cross-sectional study. Lancet Reg Health Am. 2023;17:100388.
- [CrossRef] [PubMed] [PubMed Central] [Google Scholar]
- Artificial intelligence for fracture diagnosis in orthopedic X-rays: current developments and future potential. Sicot-J. 2023;9
- [Google Scholar]
- Modern diagnostic imaging technique applications and risk factors in the medical field: a review. Biomed Res Int 2022
- [Google Scholar]
- Artificial intelligence in ct and mr imaging for oncological applications. Cancers (Basel). 2023;15:1-22.
- [Google Scholar]
- An international non-inferiority study for the benchmarking of ai for routine radiology cases : chest x-ray, fluorography and mammography. Healthcare (Basel) 2023
- [Google Scholar]
- Quality assurance of chest x-ray images with a combination of deep learning methods. Applied Sciences (Switzerland). 2023;13
- [Google Scholar]
- The role of Artificial intelligence in the assessment of the spine and spinal cord. Eur J Radiol. 2023;161
- [Google Scholar]
- Deep learning to estimate lung disease mortality from chest radiographs. Nat Commun. 2023;14:2797.
- [CrossRef] [PubMed] [PubMed Central] [Google Scholar]